Challenges in Technology, Trust, AI & Cybersecurity
On April 17, 2021, a Tesla Model S automobile, equipped with autopilot control, missed a turn and crashed into a tree at high speed, killing both passengers.[1]B. Pietsch, “2 Killed in Driverless Tesla Car Crash, Officials Say,” NYTimes.com, 2021. [Online]. Available: https://www.nytimes.com/2021/04/18/business/tesla-fatal-crash-texas.html. [Accessed: … Continue readingThe vehicle caught fire and the batteries reignited multiple times, making for massive and time-consuming efforts to extinguish the blaze. Each accident involving a vehicle with a significant degree of automation diminishes the collective level of trust in the car and the technologies used for safety and security measures.
Devices and mechanisms, in which physical components and software are integrated, are called cyber-physical systems. Self-driving cars are a prototypical cyber-physical system. Other examples include industrial robots, drones, precision farming machinery, pipelines, and many types of manufacturing and warehousing equipment. Many cyber-physical systems in use and under design and development today operate with considerable autonomy. Many types of integrated sensors, microprocessors, actuators, controllers and communication networks are utilized. Artificial intelligence (AI) methodologies are often employed, including machine learning, which easily leads to people questioning the trustworthiness of cyber-physical systems. There are many concepts related to trustworthiness, including cybersecurity, reputation, risk, reliability, belief, conviction, skepticism and assurance. Actions of betrayal and deception also undermine trust.
We explore the role of trust and cybersecurity as it applies to self-driving cars. Given the prominence in our society of cybersecurity threats, such as stolen identities, fraud, system crashes and disruptions to critical infrastructure, people easily understand that system failures can and do occur in devices, and that hackers could remotely take control of a self-driving car and deliberately cause a mishap or serious accident. With AI systems increasingly replacing human decision-making, there are serious issues concerning whether the control system can successfully match or exceed the effectiveness of human decision-making under all circumstances, especially while autonomous controls are driving on a public roadway.
Below, we present issues regarding trust, cybersecurity and AI that apply to self-driving cars, which are important and of broad interest to society.
Technology in Self-Driving Cars
Multiple technological systems must work together cooperatively to accomplish automated driving. There are many types of sensors needed to detect the conditions surrounding the car and feed data to the control systems. Then actuators, which are devices that respond to incoming sensor information, carry out controls and actions. Both internal and external communication networks provide the means for the devices to coordinate their work.
The technologies used to provide information in the vicinity of the vehicle include Lidar (light detection and ranging), long- and short-range radar, cameras and ultrasound sensors. Lidar uses an infrared beam to determine the distance between the sensor and an object in the vicinity of the car.[2]J. Kocić, N. Jovičić, and V. Drndarević, “Sensors and sensor fusion in autonomous vehicles,” 2018 26th Telecommunications Forum (TELFOR), pp. 420-425, 2018. These technologies make it possible to map and track positions and speeds of objects, such as traffic signs, vehicles, pedestrians, bicyclists and wildlife.[3]J. Hecht, “Lidar for Self-Driving Cars,” Optics and Photonics News, vol. 29, no. 1, pp. 26-33, 2018. Available: 10.1364/opn.29.1.000026. Lidar enables many functionalities, including lane following and collision avoidance. Image processing and other algorithms classify objects and help the vehicle react appropriately to hazards.
Vehicles utilize both internal and external communication networking. Internal networks employ a bus technology, which provides an electronic backbone that connects many types of devices, with specialized protocols that enable great flexibility for interconnecting the many devices within the vehicle. External connectivity is also essential, for purposes such as receiving software updates; obtaining real-time traffic information from roadside units; providing remote access for remotely monitoring, controlling climate and locking/unlocking the car; and accessing the web.
All these components have failure rates and are vulnerable to malicious intrusions that can jeopardize vehicle performance and compromise safety.
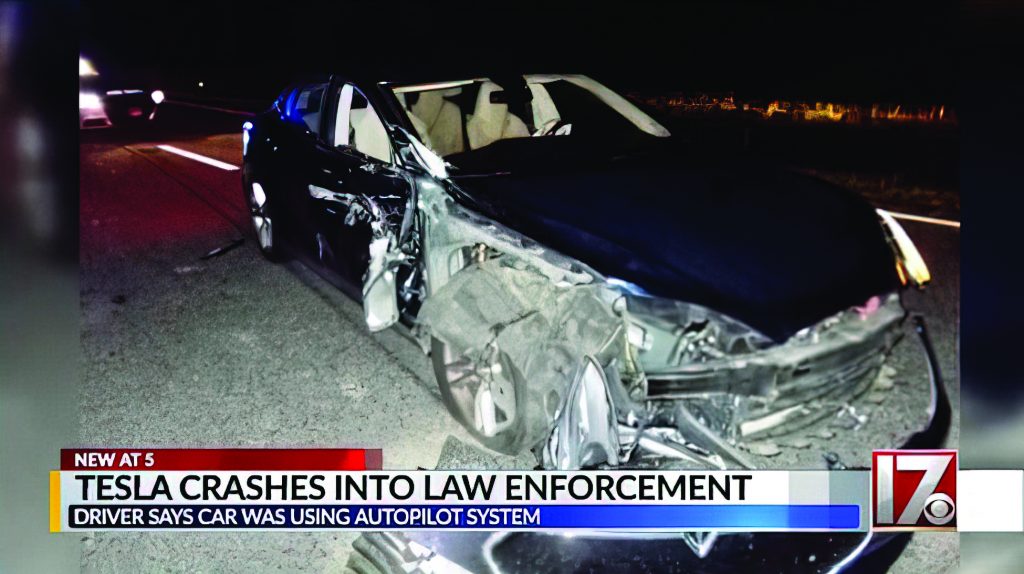
Trust
Trust is a two-sided social construct involving a trustor and a trustee. Whenever a trustor delegates a task or in some way interacts with a trustee, they make an associated decision about trust. When the trustee is a self-driving car enabled with AI methodologies, a passenger is a trustor who chooses and believes that the car will reliably and safely bring him or her to the destination. While trust refers to a relationship between trustor and trustee, trustworthiness is a property only of the trustee. A self-driving car can be deemed trustworthy if it has high performance levels in terms of categories such as competence, integrity, low risk, high reliability, high ethical standards and predictability.
There have been multiple accidents involving Tesla vehicles.[4]F. Lambert, “A fatal Tesla Autopilot accident prompts an evaluation by NHTSA – Electrek,” Electrek, 2016. [Online]. Available: … Continue reading[5]F. Lambert, “Tesla Model 3 driver again dies in crash with trailer, Autopilot not yet ruled out – Electrek,” Electrek, 2019. [Online]. Available: … Continue reading[6]G. Røed, “Tesla på auto-styring da mann ble meid ned,” Motor.no, 2020. [Online]. Available: https://motor.no/autopilot-nyheter-tesla/tesla-pa-auto-styring-da-mann-ble-meid-ned/188623. … Continue reading A Tesla car in Autopilot mode (Tesla’s self-driving software) is supposed to have a person in the driver’s seat ready to take control if needed. However, a recent video and report, released by Consumer Reports, shows that buckling the seatbelt across an empty seat and placing a weighted chain on the steering wheel can fool the vehicle into inferring that the vehicle has a driver in place.[7]L. Kolodny, “Tesla cars can drive with nobody in the driver’s seat, Consumer Reports engineers find,” cnbc.com, 2021. [Online]. Available: … Continue reading Such reports and continued accidents diminish trust in the technology and raise serious safety and security questions.
Evaluating the trustworthiness of a self-driving vehicle by a trustor is based on evidence. An important source of this evidence is reputation, which concerns reports, impressions and reviews that are available from various sources. Social media and testing laboratories are rife with such reports. Reputation sources are often consulted before people purchase a product online, an approach that readily transfers into evaluating the trustworthiness of a vehicle. It is widely held that repeated good reputation reports build trustworthiness slowly, and bad reports diminish trustworthiness quickly.
Humans are also emotional and complex beings, which means that beliefs, purpose, motivation, integrity, intentions and commitment to outcomes all play a role in trust among people. Trust between humans and a machine differs because behaviors and actions driven by these elements are difficult to program.
Artificial Intelligence (AI)
There is a fundamental question of whether a vehicle operating fully autonomously can possibly function and respond intelligently in all driving situations. Throughout the now long history of designing, developing and deploying higher technology in vehicles, each innovation has been initially met with skepticism and limited trust. A few examples include the automatic transmission, automatic braking, cruise control and electronic stability control. What these ever more sophisticated technologies have in common is that they automatically assist driving and enhance safety and comfort by monitoring and controlling conditions within the driving environment and the actions and performance of the vehicle and the driver. There is a fundamental question of whether it is possible for a vehicle operating fully autonomously to relinquish control to a human driver in standby mode in a meaningful way.
AI refers to the ability of a machine to perform tasks commonly associated with intelligent beings.[8]B. Copeland, “artificial intelligence | Definition, Examples, and Applications,” Encyclopedia Britannica, 2020. [Online]. Available: https://www.britannica.com/technology/artificial-intelligence. … Continue reading AI methodologies are broadly classified into symbolic and sub-symbolic approaches. When applied to self-driving cars, a symbolic approach directly encodes precisely what the vehicle does under a given circumstance. There can be a detailed attempt to utilize available information from sensing devices to drive program code that models intelligent actions.
By contrast, a sub-symbolic approach is based upon developing responses from experience, much as a child learns from being exposed to his or her surroundings. Machine learning is a specific type of sub-symbolic AI that can learn from examples of human behavior and mimic what a person might do. In the race to roll out a reliable and trustworthy self-driving car, automobile manufacturers are developing better and better machine learning methods that can emulate collective human behaviors at their best.
In the march toward fully automated driving, there is a debate regarding whether it is desirable to emulate human decision-making, which is known to often be flawed, or to configure systems that employ AI methodologies that can outperform the best human driver. Elon Musk has stated that a self-driving Tesla might already be smarter than a human driver.
Cybersecurity
Trust and trustworthiness are related to cybersecurity. The well-known cybersecurity triad has dimensions of confidentiality, integrity and authentication. Confidentiality refers to the protection of data and resources from unauthorized access, usage and modification. Integrity refers to the protection of data and resources from unauthorized modification, while also ensuring that the data remains accurate and reliable. Authentication refers to the accessibility of the systems and resources only to authorized users.
Compromise of any of the cybersecurity dimensions erodes trust between a trustor and trustee and diminishes trustworthiness. An autonomous vehicle that is highly vulnerable to a cybersecurity attack will have a low measure of trustworthiness. A malware attack that affects the sensing mechanisms, internal networks, controls, AI functions and computational procedures of the vehicle, while in a full or partial autonomous mode, can easily result in unpredictable and dangerous vehicle actions. Furthermore, intrusive malware often lies dormant and does its damage when a specific trigger occurs, possibly in a coordinated and synchronized way.
The illustrations on the following pages illustrate several types of attacks to which vehicles are vulnerable, all of which present risks and serve to diminish trust and trustworthiness when they occur.
There are many other types of attacks, but the ones described on the next two pages are quite common.
Most of the attacks threatening autonomous vehicles can be mitigated by the application of intelligent intrusion detection strategies for monitoring and analyzing the data packets exchanged among vehicles and road-side infrastructure. Intrusion detection methodologies are broadly categorized as Anomaly-based Detection and Signature-based Detection. Anomaly-based methods seek out abnormalities in message traffic. Signature-based detection methods are equipped with characteristics of known threat patterns and analyze incoming messages for any matches.
Trust is closely coupled with the public’s perceptions of automation. Each accident feeds perceptions that self-driving cars are disastrous and untrustworthy. Details of vehicle-crash statistics, which are available from TeslaDeaths.com, reveal that some accidents occur while the car is in Autopilot mode but are not entirely caused by the automated operation of the vehicle. For example, there were travel conditions that made an accident unavoidable, as well as incidents in which a passenger’s intervention—as when a passenger took back control of the vehicle—was the primary cause of an accident.
Social impacts of autonomous systems, on the positive side, include reducing traffic congestion, improving safety, making many human tasks easier and more convenient, and creating new jobs. On the negative side, jobs such as truck driving, one of the most common occupations, could be eliminated. Also, knowing that vehicles can be wirelessly hacked from a distance reduces trust. In July 2020, cybersecurity researchers Charlie Miller and Chris Valasek hacked into a Jeep Cherokee’s Uconnect computer from more than 10 miles away. Once they wirelessly gained control, they were able to access dashboard functions, brakes, transmission and steering.[9]S. Lawless, “Carhacked! (9 Terrifying Ways Hackers Can Control Your Car),” Purple Griffon, 2020. [Online]. Available: … Continue reading
Sybil Attack
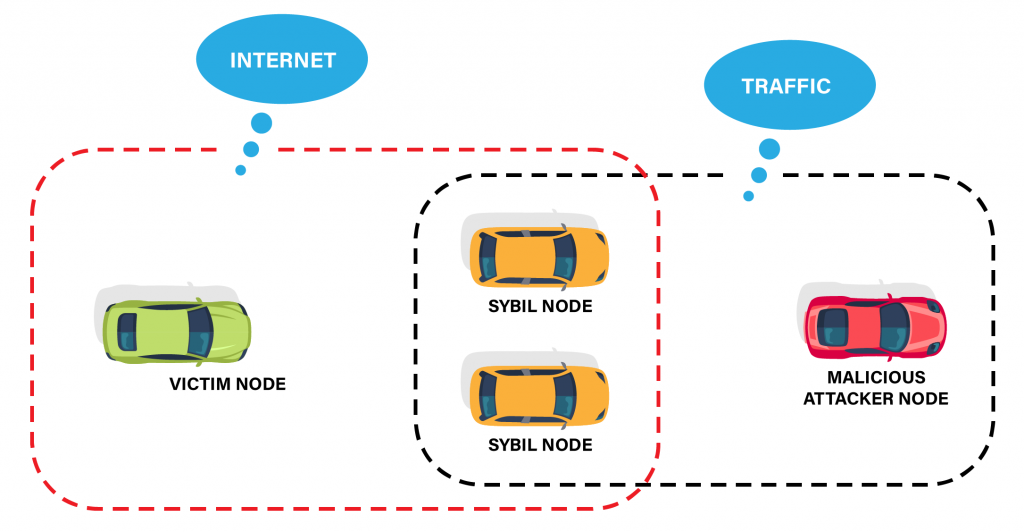
Black Hole Attack
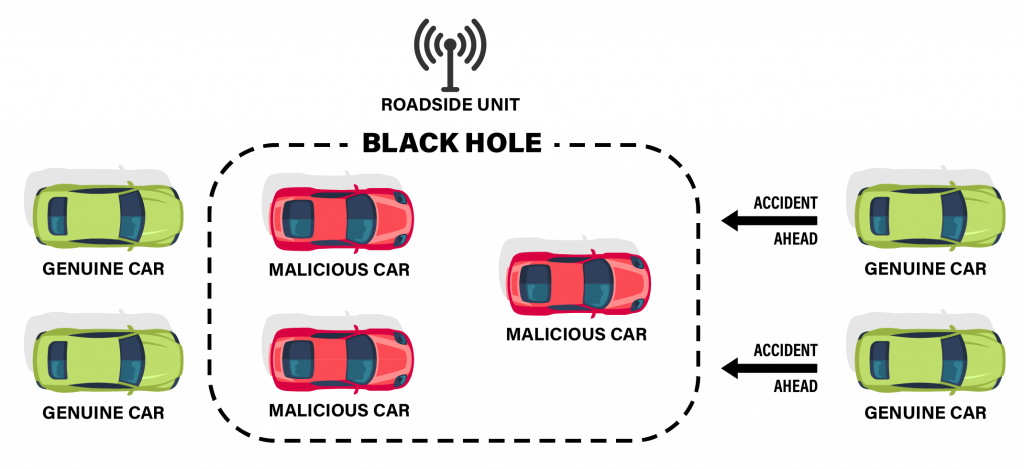
Worm Hole Attack
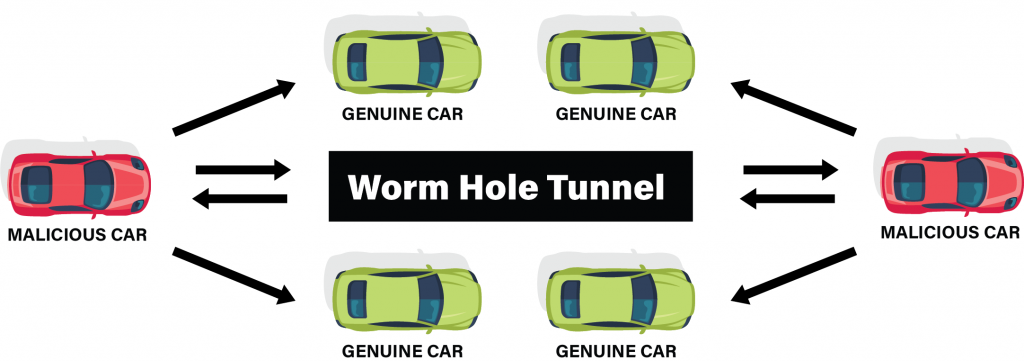
Denial-of-Service Attack
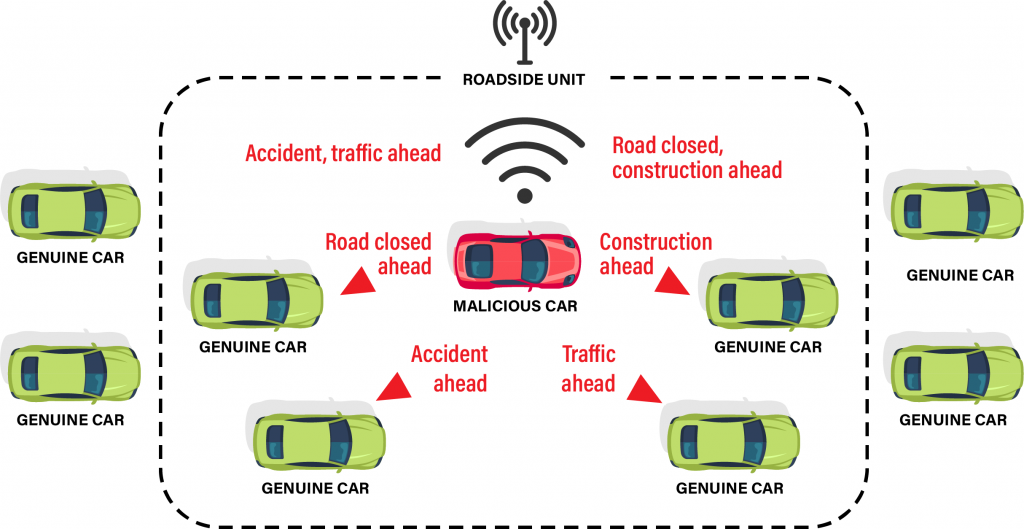
There have been instances in which human drivers—and not to mention, pedestrians and bicyclists—have tricked the internal mechanics of self-driving vehicles, causing them to crash. Last but not the least, even when vehicle manufacturers employ white-hat hackers to decode every possible hacking attack to help build a secure vehicle, there are still very smart hackers out there capable of finding that one loophole vulnerable to a hack, which can lead to a crash.
TeslaDeaths.com maintains and presents a comprehensive record of all fatalities caused by Tesla, including crash data on the geographic locations of accidents and instances where Autopilot was engaged prior to accidents, as well as crash analysis and the claims made by Tesla officials. As of May 2021, six of the 181 reported Tesla deaths were Autopilot fatalities.[10]“TeslaDeaths.com: Digital record of Tesla crashes resulting in death,” TeslaDeaths.com, 2021. [Online]. Available: https://www.tesladeaths.com/. [Accessed: May 13, 2021]. In August, the National Highway Traffic Safety Administration launched an investigation into Tesla’s Autopilot driving system.
To some extent, car drivers are actors within social settings. Characteristics that are fundamentally human, such as mercy and empathy, can influence their behaviors and actions during a crisis. In contrast, the programming and controls of a self-driving car are unlikely to have comparable characteristics, even if training is done with emulation based upon real human responses. Accidents and crash reports, involving vehicles driven in autonomous mode, reveal that an autonomous vehicle is not capable of mercy, which evokes an instinctive fear and antagonism undermining trust. Major questions remain concerning the best course of action for a car under autonomous control to follow in difficult circumstances, especially if available information is incomplete, uncertain or biased.
Promotions of autonomous vehicle technology by the automobile manufactures paint a positive picture of self-driving cars, depicting them as robust, trustworthy, economical and congestion alleviating. This article provides a service by describing and increasing awareness of a myriad of trust and trustworthiness issues and challenges surrounding self-driving cars. While acknowledging the benefits of automated vehicles, this article points out sources of risk that can undermine trust and illustrates the need for further advances and maturing of the technologies, particularly in supporting intelligent decision-making.
Aakanksha Rastogi, PhD, earned an MS and PhD in Software Engineering at North Dakota State University. She has six years of industry experience in software development and quality assurance. Currently, she works as a senior software engineer at Medtronic PLC. Dr. Rastogi has expertise and research interests in visualization methodologies, cybersecurity, machine learning and threats to autonomous systems, such as self-driving cars. She has published several conference proceedings and journal articles in notable publications, such as ICJA (International Journal of Computer Applications), ISCA (International Society for Computers and their Applications), CATA (International Conference on Computers and Their Applications) and the 2017 World Congress in Computer Science, Computer Engineering & Applied Computing. Dr. Rastogi co-authored a book chapter in Advances in CyberSecurity Management, published by Springer. She has volunteered in staffing summer workshops in cybersecurity for middle and high school girls.
Kendal E. Nygard, PhD, is the Director of the Dakota Digital Academy and Emeritus Professor of Computer Science at NDSU where he joined the faculty in 1977 and served as department chair for 12 years. His many accomplishments include operations research at the Naval Postgraduate School, a research fellowship at the Air Vehicle Directorate of the Air Force Research Lab. In 2013-14, he had the distinctive honor of serving as a Jefferson Science Fellow at the US Agency for International Development in Washington, DC.
References
↑1 | B. Pietsch, “2 Killed in Driverless Tesla Car Crash, Officials Say,” NYTimes.com, 2021. [Online]. Available: https://www.nytimes.com/2021/04/18/business/tesla-fatal-crash-texas.html. [Accessed: May 9, 2021]. |
---|---|
↑2 | J. Kocić, N. Jovičić, and V. Drndarević, “Sensors and sensor fusion in autonomous vehicles,” 2018 26th Telecommunications Forum (TELFOR), pp. 420-425, 2018. |
↑3 | J. Hecht, “Lidar for Self-Driving Cars,” Optics and Photonics News, vol. 29, no. 1, pp. 26-33, 2018. Available: 10.1364/opn.29.1.000026. |
↑4 | F. Lambert, “A fatal Tesla Autopilot accident prompts an evaluation by NHTSA – Electrek,” Electrek, 2016. [Online]. Available: https://electrek.co/2016/06/30/tesla-autopilot-fata-crash-nhtsa-investigation/. [Accessed: May 9, 2021]. |
↑5 | F. Lambert, “Tesla Model 3 driver again dies in crash with trailer, Autopilot not yet ruled out – Electrek,” Electrek, 2019. [Online]. Available: https://electrek.co/2019/03/01/tesla-driver-crash-truck-trailer-autopilot/. [Accessed: May 9, 2021]. |
↑6 | G. Røed, “Tesla på auto-styring da mann ble meid ned,” Motor.no, 2020. [Online]. Available: https://motor.no/autopilot-nyheter-tesla/tesla-pa-auto-styring-da-mann-ble-meid-ned/188623. [Accessed: May 9, 2021]. |
↑7 | L. Kolodny, “Tesla cars can drive with nobody in the driver’s seat, Consumer Reports engineers find,” cnbc.com, 2021. [Online]. Available: https://www.cnbc.com/2021/04/22/tesla-can-drive-with-nobody-in-the-drivers-seat-consumer-reports.html. [Accessed: May 9, 2021]. |
↑8 | B. Copeland, “artificial intelligence | Definition, Examples, and Applications,” Encyclopedia Britannica, 2020. [Online]. Available: https://www.britannica.com/technology/artificial-intelligence. [Accessed: May 9, 2021]. |
↑9 | S. Lawless, “Carhacked! (9 Terrifying Ways Hackers Can Control Your Car),” Purple Griffon, 2020. [Online]. Available: https://purplegriffon.com/blog/carhacked-9-terrifying-ways-hackers-can-control-your-car. [Accessed: May 13, 2021]. |
↑10 | “TeslaDeaths.com: Digital record of Tesla crashes resulting in death,” TeslaDeaths.com, 2021. [Online]. Available: https://www.tesladeaths.com/. [Accessed: May 13, 2021]. |