The invention of useful artificial intelligence (AI), epitomized by the hype over ChatGPT, is the latest example of a basic truth about technology. There have always been many more inventions that use energy than those that can produce it. Such is the nature of progress in all domains from medicine and entertainment to information and transportation. While there’s a lot of debate, and angst, about AI’s implications for the economy, jobs and even politics, there’s no debate about the fact that it is a big deal and applications for using it are growing at a blistering pace. It’s obvious, but worth stating, that the invention of the car, for example, also ‘invented’ demand for energy to build and operate cars. Now, in the still short history of silicon chips—the engines of the information age—the arrival of AI promises an unprecedented boost to future energy demand.
Consider an analogy. Imagine it’s 1925 and there was a global conference that convened internal combustion engineers.
At that point in history, combustion engines and cars had been around for almost four decades, and engines had become good enough in the previous decade to enable building aircraft. Also imagine a Ford engineer at that conference forecasting engine progress that would soon make it practical for widespread air travel. (Yes, a Ford engineer because that company pioneered not only aviation engines, but one of the first practical passenger aircraft, the 1925 TriMotor.i” And imagine if that engineer had suggested that global air traffic would become so common that aviation in the foreseeable future would consume nearly as much energy as the entire U.S. used in 1925 for all purposes. A year later, 1926, saw the launch of America’s first regularly scheduled, year-round commercial passenger service. The rest, as they say, is history. Fast forward to an actual conference in 2022—one that took place several months before the November unveiling of ChatGPT—where the CTO of AMD, a world-leading AI chipmaker, talked about how powerful AI engines have become and how fast they were getting adopted. He also showed a graph forecasting that by 2040, AI would consume roughly as much energy as the U.S. does today for all purposes.ii If those trends continued, AI would end up gobbling up most of the world’s energy supplies. Of course, the trends won’t continue that way, but that doesn’t change the fact that, as another engineer at AI chipmaker ARM said at that conference: “The compute demand of [AI] neural networks is insatiable.” And the growth of AI is still in the early days, equivalent to aviation circa 1925 or, in computer-history terms, to the pre-desktop 1980s era of mainframes.
Inference as Efficient Energy Hog
It’s not news to the computer engineering community that AI has a voracious energy appetite. AI-driven “inference,” rather than conventional “calculation,” is the most power-intensive use of silicon yet created.iii That reality is starting to leak out because of the popularity of today’s first-generation AI. We see headlines about AI having a “booming … carbon footprint”iv and that it “guzzles energy.”v
Consider, for example, the results of one analysis of what it takes to just build, never mind operate, a
modest AI tool for one application, i.e., the equivalent of the energy used to build an aircraft, not fly it. The analysis found that the training phase—training is how an AI tool is built—consumed more electricity than the average home does in 10 years.vi A different analysis looked at what it likely took to build a bigger AI tool, say to train ChatGPT, finding that it used as much electricity as an average home in a century.vii Of course, like automobiles and aircraft, energy is also used to operate AI, something engineers call “inference,” to, say, recognize an image or an object, or give advice or make a decision, etc. Energy use by inference is likely tenfold or more greater than for training.viii As one researcher put it, “it’s going to be bananas.”
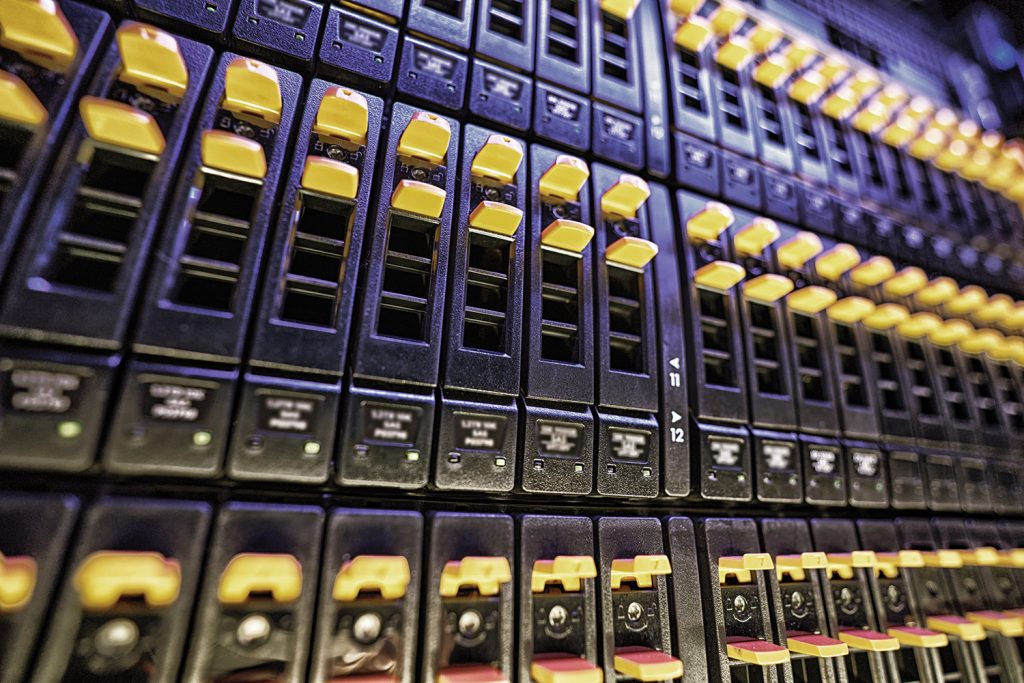
AI tools like ChatGPT are costly to train, both financially, due to the cost of hardware and electricity, and environmentally, due to the carbon footprint required to fuel data processing hardware.
AI as General Purpose Tech
There are few intersections of the world of bits and atoms—of software and hardware—that so dramatically epitomize the inescapable realities of the physics of energy, and the challenges in guessing future behaviors and thus energy demands. No one can guess the number of important, or trivial, things where we will want to use AI. And, in fact, for most people in the (lucky) wealthy nations, most energy is used for things other than mere survival. Aviation, for example, is dominated by citizens traveling for fun, vacations, to see family; business travelers account for well under 20 percent of global air-passenger miles (even less now, during the recovery period from global lockdowns). However, unlike aircraft, which are specialized machines used to move goods and people, AI is a universal tool, a “general purpose technology” in the language of economists, and thus has potential applications in everything, everywhere. It’s far harder to forecast uses of general-purpose technologies.
AI tools will be put to work for much more than just fine-turning advertising, or performing social media tricks, or creating “deepfakes” to spoof hapless citizens, or making self-driving cars (eventually) possible. AI’s power and promise, as its practitioners know, are leading to the potential for such applications as hyper-realistic vehicle crash-testing, or monitoring and planning ground and air-traffic flows, or truly useful weather forecasting. The most profound applications are to be found in basic discovery wherein AI-infused supercomputers plumb the depths of nature and simulate molecular biology “in silico” (an actual term), instead of in humans in order to both accelerate discovery and even, eventually, to test drugs. The number and nature of potential applications for AI is essentially unlimited.
Datacenters & Infrastructure Buildout
Analysts have pointed out that the compute power— and derivatively energy—devoted to machine learning has been doubling every several months.ix Last year, Facebook noted that AI was a key driver causing a one-year doubling in its datacenter power use.x And this year, Microsoft reported a 34 percent “spike” in water used to cool its datacenters, an indirect even if unstated measure of energy use.xi It’s the equivalent of measuring the flow of water to cool a combustion engine rather than counting gallons of fuel burned. The need for cooling comes from the energy use.
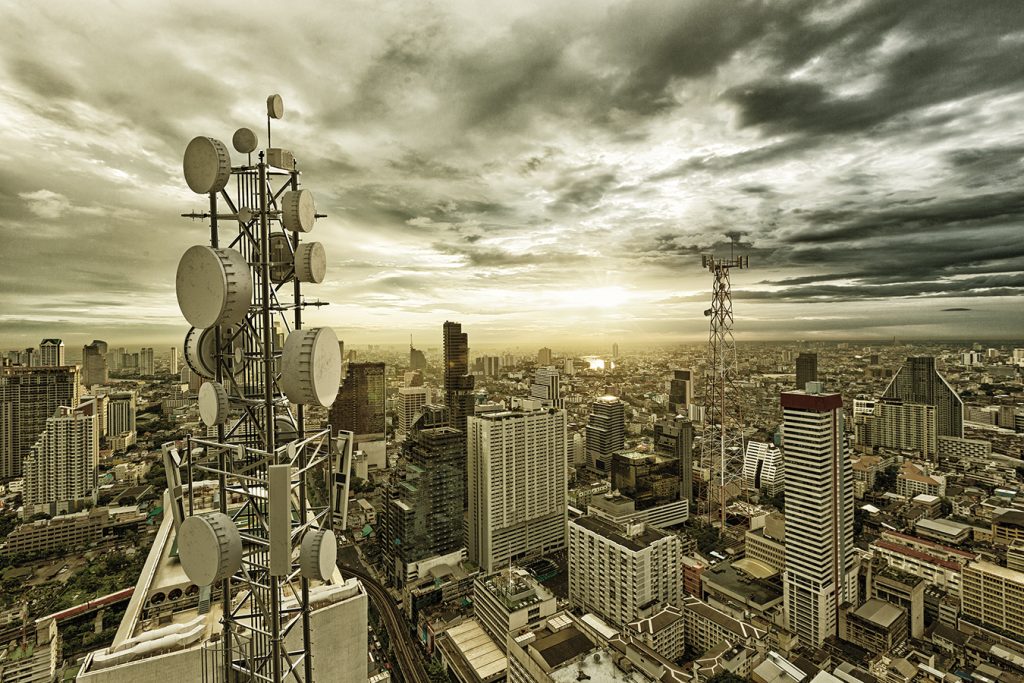
It is an open secret that AI will drive a massive infrastructure buildout. As a Google VP observed, deployment of AI is “really a phase change in terms of how we look at infrastructure.” One article’s headline captured the reality: “The AI Boom Is here. The Cloud May Not Be Ready.”xii Every computer vendor, chipmaker, software maker and IT service provider is adding or expanding offerings that entail AI. It is a silicon gold rush last matched in enthusiasm and velocity during the great disruption in information systems of the 1990s with the acceleration from mainframes to desktops and handhelds. AI enthusiasm is also seen in the stock market where, odds are, history will repeat as well: a boom, a bust and then the long boom. It’s the coming long boom that has implications for forecasting energy demands from AI.
To continue with our analogy, while infrastructure growth points to potential for future energy use, it doesn’t predict actual outcomes any more than counting highway or runway miles is predictive of fuel use, except that the infrastructure is what enables the fuel to be used. Future historians will see today’s Cloud infrastructure as analogous to the 1920s stage of transportation infrastructure in the pre- superhighway days, also a time of grass runways. Even before we see what the next phase of silicon evolution brings—the kinds of services and social changes that will echo those brought by the automobile and aviation—it’s possible to have some idea of the scale of energy demand that will bring by considering the current state of today’s information infrastructure.
Unlike automobiles and aircraft though, the energy used by digital engines is hidden from plain sight inside thousands of nondescript warehouse-like datacenters. There we find, in each one of them, thousands of refrigerator-sized racks of silicon machines. Each such rack burns more electricity annually than 15 homes, that’s before the racks are filled with AI silicon.
Datacenters, in square footage terms, are the skyscrapers of the modern era, except that there are far more of the former and many more being built. And each square foot of datacenter uses 100 times the power of a square foot of skyscraper—again, before the infusion of AI.
Datacenters of course are only useful if connected to people and other machines and vice versa. The world today has over a billion miles of information highways comprised of glass cables along with four million cell towers that forge an invisible, virtual highway system that is effectively another 100 billion miles long.xiii Machinery for transporting bits uses energy just as it does in the world of atoms. And the convenience of wireless networks comes with an energy cost up to 10 times more energy per byte,xiv not unlike a similar principle of physics that makes flying more fuel-intensive than driving. Flight-shamers might take note: In energy-equivalent terms, today’s global digital infrastructure already uses roughly 3 billion barrels of oil annually, rivaling the energy used by global aviation. And that number is based on data that is a half-dozen years old. Since then, there’s been a dramatic acceleration in datacenter spendingxv on hardwarexvi and buildingsxvii along with a huge jumpxviii in the power density of that hardware, all of that, again, before the acceleration as AI is added to that infrastructure. A single, simple AI-driven query on the Internet can entail over fourfold the energy use of a conventional query.xix
It’s not that digital firms are energy wastrels. In fact, silicon engineers have achieved epic, exponential gains in efficiency. But overall demand for logic has grown at an even faster, blistering pace. You can “take to the bank” that history will repeat here too: demand for AI services will grow faster than improvements in AI energy efficiency. The cloud is already the world’s biggest infrastructure and seeing it expand yet by several-fold, or more, would be entirely in keeping with historical precedent.
Jevons Paradox
Nonetheless, the forecasters and pundits who are preoccupied with reducing society’s energy appetite always offer energy efficiencyxx as a “solution” to the energy “problem.” They have it backwards. Efficiency gains have always been the engine that drives a growth, not a decrease, in overall energy use. It’s a feature, not a bug, in technology progress, and one that is most especially true in digital domains. This seeming contradiction has been called Jevons Paradox after the British economist William Stanley Jevons who first codified the economic phenomenon of efficiency in a seminal paper published back in 1865. That paper was focused on the claim, at that time, that England would run out of coal given the demands for that fuel coming from a growing economy, growth that itself was caused by the fuel of industrialization. The solution offered by experts at that time was to make coal engines more efficient.
Jevons, however, pointed out that improvements in engine efficiency—i.e., using less coal per unit of output—would cause more, not less, overall coal consumption. Thus, the ostensible paradox: “It
is wholly a confusion of ideas to suppose that the [efficient] use of fuel is equivalent to a diminished consumption …. new modes of [efficiency] will lead to an increase of consumption.”xxi Some modern economists call this the “rebound effect.”xxii It’s not a rebound as much as it’s the purpose of efficiency.
Put differently: the purpose of improved efficiency in the real world, as opposed to the policy world, is to make it possible for the benefits from a machine or a process to become cheaper and available to more people. For nearly all things for all of history, rising demand for the energy-enabled services outstrips the efficiency gains. The result has been a net gain in consumption.
If affordable steam engines had remained as inefficient as when first invented, they would never have proliferated, nor would the attendant economic gains and associated rise in coal demand have happened. The same is true for modern combustion engines. Today’s aircraft, for example, are three times more energy efficient than the first commercial passenger jets. That efficiency didn’t “save” fuel but instead propelled a four-fold rise in aviation energy use.xxiii
The same dynamic is at play with today’s digital engines, the driving force of the 21st-century economy. In fact, the microprocessor represents the purest example of the Jevon’s paradox. Over the past 60 years, the energy efficiency of logic engines has improved by over one billion fold.xxiv Nothing close to such gains are possible with mechanical and energy machines that occupy the world of atoms.
Consider the implications from 1980, the Apple II era. A single iPhone at 1980 energy-efficiency would require as much power as a Manhattan office building. Similarly, a single datacenter at circa 1980 efficiency would require as much power as the entire U.S. electrical grid. But because of efficiency gains, the world today has billions of smartphones and thousands of datacenters. We can only hope and dream that the efficiency of AI engines advances similarly.
A leading-edge AI chip today delivers more image processing capability than a supercomputer could just two decades ago. In a sign of our times, last year the silicon start-up Cerebras introduced a kind of Godzilla-class AI chip the size of an entire silicon wafer (think, medium pizza) with more than two trillion transistors and a 15-kilowatt power appetite. That’s more peak power than used by three houses. But it offers more than a ten-fold gain in efficiency over the best AI chips. Competitors will follow. That’s why the market for AI chips is forecastxxv to dominate semiconductor growth and explode some 700 percent in the next five years alone.
Green vs AI
Of course, the Jevons paradox breaks down in a microeconomic sense, and for specific products or services. Demand and growth can saturate in a (wealthy) society when limits are hit for specific items regardless of gains in efficiency, e.g., the amount of food a person can eat, or the miles-per-day one is willing to spend driving, or the number of refrigerators or light bulbs per household, etc. But for such things, we’re a long way from saturation for over two-thirds of the world’s citizens. Billions of people in the world have yet to become wealthy enough to buy even their first car or air conditioner, never mind use an AI-infused product or service.
But one can understand why the “green AI” community is alarmed over what will come. Even before the Age of AI is in full swing, today’s digital infrastructure already uses twice as much electricity as the entire country of Japan. We await the Cloud forecasts that incorporate the energy impact of the AI gold rush. As Deep Jariwala, a professor of electrical engineering at the University of Pennsylvania provocatively put it: “By now, it should be clear that we have an 800-pound gorilla in the room; our computers and other devices are becoming insatiable energy beasts that we continue to feed.”xxvi Prof. Jariwala went on to caution: “That’s not to say AI and advancing it needs to stop because it’s incredibly useful for important applications like accelerating the discovery of therapeutics.” There’s little to no risk that governments will or can directly throttle AI development (though some have proposed as much). Ironically though, government energy policies could make AI expensive enough to slow deployment.
Consider a simple arithmetical reality: In a low-cost state, training a high-end AI requires buying about $100,000 in electricity, but you’d spend over $400,000 in California. And the training phase for many applications is necessarily repeated as new data and information are accumulated. One can imagine, as some have proposed, doing the training at remote locations where electricity is cheap. That’s the equivalent of, say, buying energy-intensive aluminum to build airplanes from places where energy is cheap (China, using its coal-fired grid, produces 60 percentxxvii of the world’s aluminum). But most uses for AI require operating it and fueling it locally and in real-time, much as is the case to operate an airplane. Wealthy people will be able to afford the benefits from higher cost AI services, but that trend will only further widen the “digital divide,” wherein lower income households are increasingly left behind.
Transition Irony
There is some irony in fact that many in the tech community have joined with the “energy transition” lobby to promote the expansion of power plants that are not only increasing the cost of electricity but making it more difficult to deliver it when markets need it. Despite the mantra that wind and solar are cheaper than conventional power plants, the data show that, in every state and every country, the deployment of more episodic power leads to rising electricity costs. The reason for that, in essence, comes from the cost of ensuring that power is delivered whenever markets and people need it, and not when nature permits it. It doesn’t matter whether the reliability is achieved by maintaining what amounts to a duplicate, under-utilized existing grid (Germany’s solution), or by using use more transmission lines and more storage. The results lead to far higher costs.
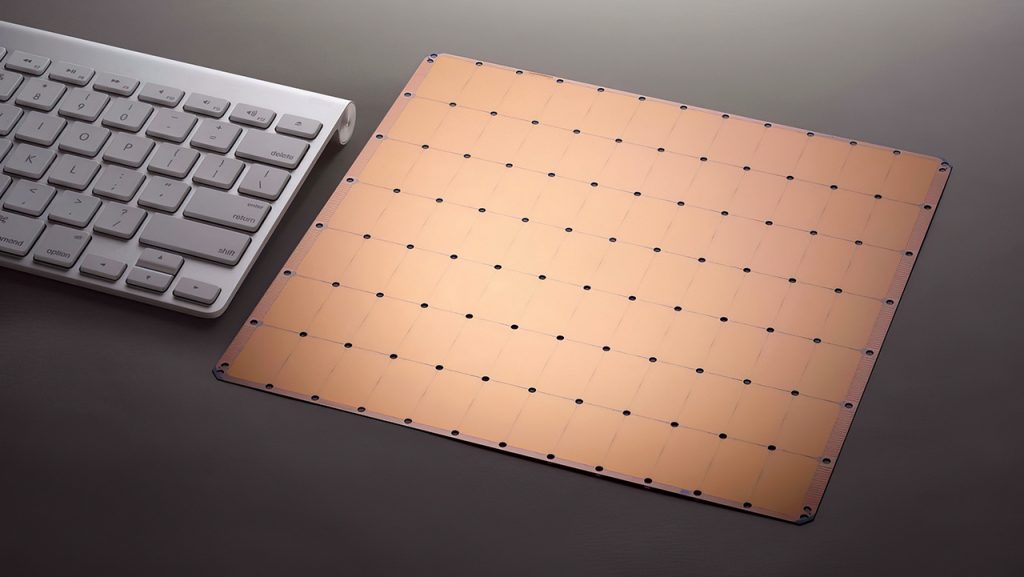
The Cerebras “wafer-scale engine,” measuring 8.5 inches on a side, is the world’s largest chip, and is dedicated to computations prevalent in machine-learning forms of artificial intelligence. Photograph / Cerebras Systems.
Policies that lead to higher costs and lower reliability for electricity will be increasingly in collision with the emerging demands for an AI-infused future. That may be the most interesting and challenging intersection of the worlds of bits and atoms. ◙
References
I https://simpleflying.com/henry-ford-aviation-pioneer-story/
ii https://semiengineering.com/ai-power-consumption-exploding/
vi https://arxiv.org/abs/1906.02243
viii https://xcorr.net/2023/04/08/how-much-energy-does-chatgpt-use/
ix https://arxiv.org/pdf/1907.10597.pdf
x https://www.eetimes.com/qualcomm-targets-ai-inferencing-in-the-cloud/
xi https://fortune.com/2023/09/09/ai-chatgpt-usage-fuels-spike-in-microsoft- water-consumption/
xii https://www.wsj.com/articles/the-ai-boom-is-here-the-cloud-may-not-be- ready-1a51724d
xvi https://www.nextplatform.com/2019/12/09/datacenters-are-hungry-for-servers-again/
xvii https://www.construction.com/construction-news/
xix https://www.nytimes.com/2023/04/16/technology/google-search-engine-ai.html
xxi Jevons, William Stanley, The Coal Question, Macmillan and Co., 1865. https://www.econlib.org/library/YPDBooks/Jevons/jvnCQ.html
xxii Nordhaus, Ted. “The Energy Rebound Battle.” Issues in Science and Technology, July 28, 2020. https://issues.org/the-energy-rebound-battle/
xxiii Larkin, Alice, Kevin Anderson, and Paul Peeters. “Air Transport, Climate
Change and Tourism.” Tourism and Hospitality Planning; Development 6, no. 1
(April 2009): 7–20. https://doi.org/10.1080/14790530902847012.
xxiv Roser, Max, and Hannah Ritchie. “Technological Progress.” Our World in Data, May 11, 2013. https://ourworldindata.org/technological-progress
xxv https://www.eetimes.com/iot-was-interesting-but-follow-the-money-to-ai-chips/?image_number=1
xxvi https://penntoday.upenn.edu/news/hidden-costs-ai-impending-energy-and-resource-strain
Mark Mills is a Manhattan Institute Senior Fellow, a Faculty Fellow in the McCormick School of Engineering at Northwestern University and a cofounding partner at Cottonwood Venture Partners, focused on digital energy technologies. Mills is a regular contributor to Forbes.com and writes for numerous publications, including City Journal, The Wall Street Journal, USA Today and Real Clear. Early in Mills’ career, he was an experimental physicist and development engineer in the fields of microprocessors, fiber optics and missile guidance. Mills served in the White House Science Office under President Ronald Reagan and later co-authored a tech investment newsletter. He is the author of Digital Cathedrals and Work in the Age Robots. In 2016, Mills was awarded the American Energy Society’s Energy Writer of the Year. On October 5, 2021, Encounter Books will publish Mills’ latest book, The Cloud Revolution: How the Convergence of New Technologies Will Unleash the Next Economic Boom and A Roaring 2020s.