The National Security Commission on Artificial Intelligence (NSCAI) was established by Congress in 2018 to “consider the methods and means necessary to advance the development of artificial intelligence … to comprehensively address the national security and defense needs of the United States.”i And this it did. More than 19 of the recommendations from NSCAI’s “The Final Report” were included in the FY2021 National Defense Authorization Act, with dozens of other recommendations influencing Acts of Congress and executive orders related to national defense, intelligence, innovation and competition.
Although the final report was released in March 2021—and the commission sunset in October that year—one element of the report is still widely underappreciated, especially outside of government circles. While the Department of Defense (DOD), as well as other government agencies ranging from the Department of Veteran Affairsii to the Internal Revenue Service,iii have responded to the charge to adopt AI, very few government and commercial entities have invested in the calls to secure AI.
Organizations that adopt AI also adopt AI’s risks and vulnerabilities. Intentional attacks against AI are a nascent style of cyberattack by which an attacker can manipulate an AI system. So, while the U.S. does need more investment in AI, it also needs to decisively secure industry security. The purpose of the book is to provide decision-makers with context that will sharpen their critique as they embrace the power of AI in government and industry. We also offer recommendations on how the intersection of technology, policy and law can provide us with a secure future.
Below is an excerpt from the book’s first chapter, “Do You Want to Be a Part of the Future?”
NSCAI Genesis Story
Ylli Bajraktari is not a household name, but in national security circles, he has a reputation for getting things done. Andrew Exum, a former Deputy it from its adversaries.
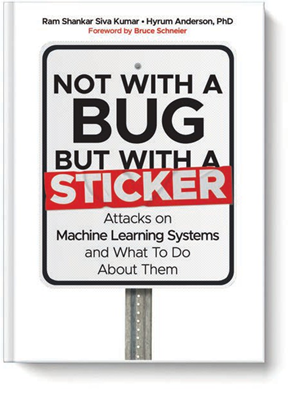
John Wiley & Sons, Inc., 2023.
In response, we wrote a book, Not With a Bug, But With a Sticker: Attacks on Machine Learning Systems and What To Do About Them, which will be published by John Wiley & Sons, Inc., on May 2, 2023. The book explains how AI systems are significantly at risk from attacks in both simple and sophisticated ways, which can jeopardize national security, as well as corporate and Secretary of Defense for the Middle East, described Bajraktari and his brother Ylberiv as “two of the most important and best people in the federal government you’ve likely never heard of.”v Ylli Bajraktari escaped war-torn Kosovo and moved to the U.S. in his 20s. Burnished with bonafide credentials from Harvard’s prestigious Kennedy School, he steadily rose through the ranks at the Pentagon, eventually becoming an advisor to the Deputy Secretary of Defense.vi
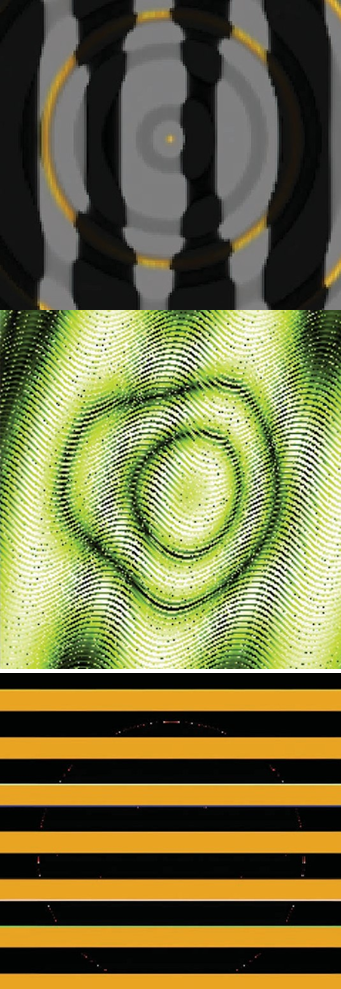
Whether it was fatigue that came from years of traveling the world to shape international policy or the pressure of working at the White House,vii Bajraktari left the executive branch to join the National Defense University’s (NDU) Institute for National Strategic Studies as a visiting research fellow. A year-long assignment from the Office of the Secretary of Defense, this break from the intensity in the White House gave him time to study and recalibrate at the NDU’s libraries. He used some rare downtime for self-education on what he thought would be an instrumental cornerstone of U.S. competitiveness: artificial intelligence (AI). Bajraktari quickly absorbed the information he gleaned from pouring over books and watching YouTube videos on machine learning (ML). While at NDU, he organized the university’s first ever AI symposium in November 2018. Expecting a meager 10 people to attend, the response was overwhelming. Bajraktari had to turn away hundreds of would-be attenders because of the room’s fire code. While Bajraktari didn’t know it yet, his time at DOD, the White House and now at NDU had been preparing him for a leadership role to shape the nation’s strategy for investing in AI.
That came in 2018, when the NSCAI was born out of the House Armed Services Committee. The leadership and guidance for the commission was to come from 15 appointed commissioners, a mix of tech glitterati that included current and former leadership at Google, Microsoft, Amazon, Oracle, and directors of laboratories at universities and research institutes that support national security.viii It was an independent, temporary and bipartisan commission set up to study AI’s national security implications.
When NSCAI commissioner and former Google CEO, Eric Schmidt, called Bajraktari to ask him to lead the commission, Bajraktari didn’t answer the phone. It was Christmas break. Plus, he had been primed by his White House days to ignore calls from unknown numbers. So eventually, the former CEO of Google resorted to the plebian tactic: He sent Bajraktari an email. In it, Schmidt described that he had just been voted by the other commissioners to chair NSCAI and needed somebody to run the commission’s day-to-day operations. Bajraktari’s email response was a simple one-liner: “I’ll do it.”ix
With an ambitious goal, a tight deadline and a budget smaller than what it takes to air a 60-second Superbowl commercial, Bajraktari assembled a team of over 130 staff members to deliver a series of reports that would culminate in NSCAI’s final report.
Even the initial findings packed a punch. Bajraktari and the two chairs of the commission headed to the White House to brief President Trump about the findings. Scheduled for 15 minutes, the meeting at the Oval Office lasted for nearly an hour. In December 2020, at the twilight of his presidency, President Trump signed an executive order entitled “Promoting the Use of Trustworthy Artificial Intelligence in Government.”x
Even with a change in the executive office, NSCAI’s recommendations continued to make an impact. On July 13, 2021—well into the Biden presidency—the commission held a summit to discuss the final report at the Mayflower Hotel Ballroom in Washington, the Secretaries of Defense, Commerce and State, the National Security Advisor, and the Director of the Office of Science and Technology Policy all made in-person appearances and spoke to the masked and socially distanced audience members. It was a powerful signal from the American government to both its allies and adversaries that the U.S., from its highest levels of trade, diplomacy, defense, security, and science and technology, was ready to invest in AI and take the NSCAI’s recommendation seriously.
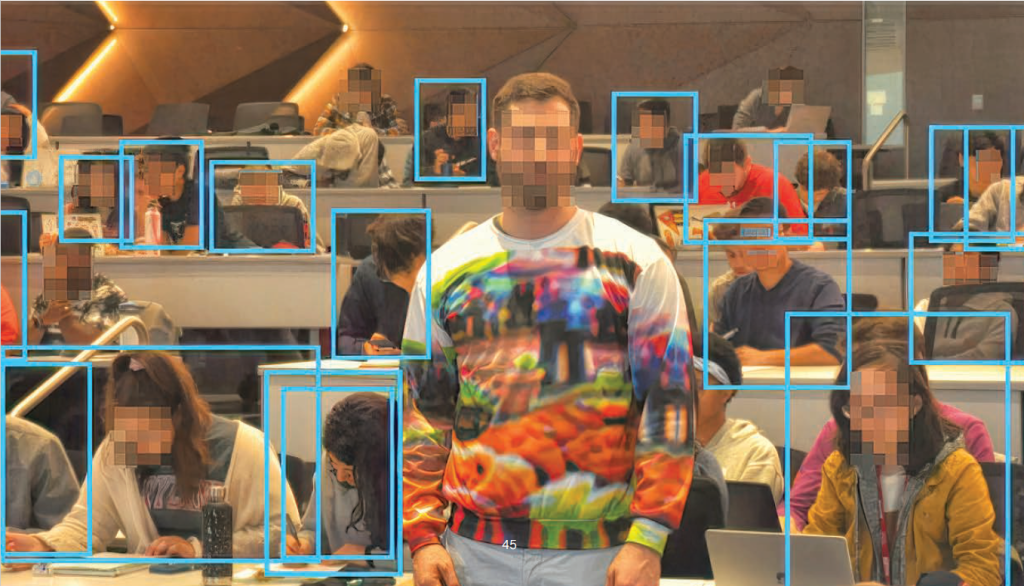
Adversarial ML: Attacks Against AI
Delivered on March 2021 to the president and Congress, the first page of NSCAI’s final report includes a realistic assessment of where the country stands, which by the commission’s own admission was uncomfortable to deliver. The NSCAI report was 756 pages long, but its opening lines summarize our unpreparedness. “America is not prepared to defend or compete in the AI era,” it reads. “This is the tough reality we must face. And it is this reality that demands comprehensive, whole-of-nation action.” To “defend … in the AI era” certainly refers to holistic national security but also entails defending vulnerabilities in AI defenses that are spelled out in numerous recommendations to ensure that “models are resilient to … attempts to undermine AI-enabled systems.”xi
But defend from whom? Defend from what?
Adversarial ML is sometimes called “counter AI” in military circles. Distinct from the case where AI is used to empower an attacker (often called offensive AI), in adversarial ML, the adversary is targeting an AI system as part of an attack. In this kind of cyberattack against AI, attackers actively subvert vulnerabilities in the ML system to accomplish their goal.
What are the vulnerabilities? What kinds of attacks are possible? Consider a few scenarios:
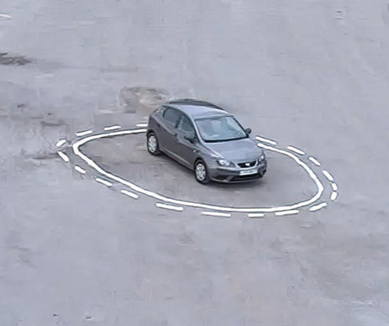
- Cyber threat actors insert out-of-place words into a malicious computer script that causes AI-based malware scanners to misidentify it as safe to run.
- A fraudster issues payment using a personal check that appears to be written for $900, but the victim’s automated bank teller recognizes and pays out only $100.
- An eavesdropper issues a sequence of carefully crafted queries to an AI medical diagnosis assistant in order to reconstruct private information about a patient that was discarded after training—but inadvertently memorized by— the AI system.
- An adversary corrupts public data on the internet used to train a facial recognition biometric authentication tool so that anyone wearing a panda sticker on their forehead is granted system access.
- A corporation invests millions of dollars to develop proprietary AI technology, but a competitor replicates it for only $2,000 by recording responses of the webservice to carefully crafted queries.
All of these scenarios—or scenarios very much like them—have been demonstrated (in some cases, by the authors) against high-end deployed, commercial models. They are all a form of adversarial ML, which is not just subversive but also subterranean in our discourse.
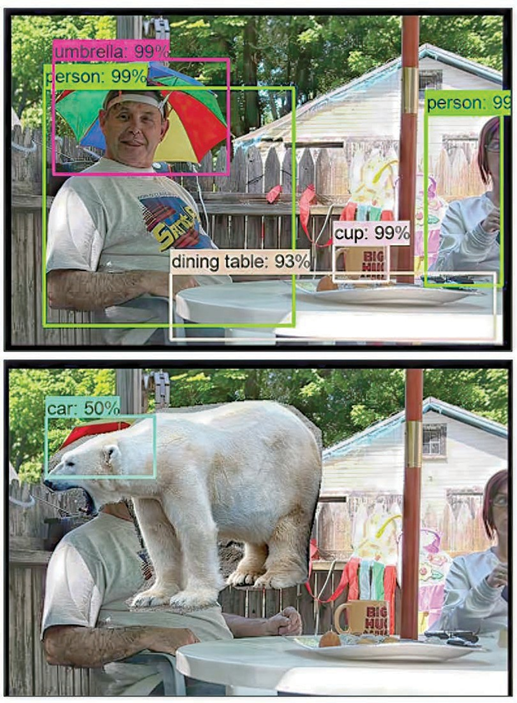
Chances are you have heard more about deepfakes (a form of offensive AI) than adversarial ML. But adversarial ML attacks are an older, but still pernicious, threat that has become more serious as governments and businesses adopt AI.
The NSCAI report wrote unequivocally about this point: “The threat is not hypothetical … adversarial attacks are happening and already impacting commercial ML systems.”
ML Systems Don’t Wobble, They Fold
To understand adversarial ML, we first need to understand how AI systems fail.
An unintentional failure is the failure of an ML system with no deliberate provocation. This happens when a system produces a formally correct but often nonsensical outcome. Put differently, in unintentional failure modes, the system fails because of its inherent weirdness. In these cases, anomalous behavior often manifests itself as earnest but awkward “Amelia Bedelia” adherence to its designers’ objectives. For instance, an algorithm that was trained to play Tetris learned how to pause the game indefinitely to avoid losing.xii The learning algorithm penalized losing, so the AI did whatever was in its power to avoid that scenario. Scenarios like this are like the Ig Nobel Prize—where it first makes you laugh and then makes you think.
But not all cases are humorous.
The U.S. Air Force trained an experimental ML system to detect surface-to-surface missiles. At first, the system demonstrated impressive 90 percent accuracy. But instead of getting a game-changing target recognition system, the Air Force learned a sobering lesson during field testing. “The algorithm did not perform well. It actually was accurate maybe about 25 percent of the time,” an Air Force official remarked.xiii It turns out that the ML system was trained to detect missiles that were only flying at an oblique angle. The accuracy of the system plummeted when the system was tested on vertically oriented missiles. Fortunately, this system was never deployed.
Unintentional failure modes happen in ML systems without any provocation.
Conversely, intentional failure modes feature an active adversary who deliberately causes the ML system to fail. It should come as no surprise that machines can be intentionally forced to make errors. Intentional failure modes are particularly relevant when one considers an adversary who gains from a system’s failure either the hidden secrets in training data memorized by the system or the intellectual property that enables the AI system to work. This branch of failures in AI systems is now generally called “adversarial machine learning.” Research in this has roots in the 1990s when considering maliciously tampered training sets and, in the 2000s, with early attempts to evade AI-powered email spam filters.
But adversary capabilities exist on a spectrum. Many require sophisticated knowledge of AI systems to pull off attacks. But one need not always be a math whiz to attack an ML system. Nor does one need to wear the canonical hacker hoodie sitting in a dark room in front of glowing screens. These systems can be intentionally duped by actors of varying levels of sophistication.
The word “adversary” in adversarial machine learning instead refers to its original meaning in Latin, adversus, which literally means someone who “turns against”— in this case, the assumptions and purposes of the AI system’s original designers. When ML systems are built, designers make certain assumptions about the place and manner of the system’s operation. Anyone who opposes these assumptions or challenges the norms upon which the ML model is built is, by definition, an adversary.
Take the event held by the Algorithmic Justice League, a digital advocacy nonprofit founded by Joy Buolamwini, as an example. In 2021, the nonprofit held a workshop called “Drag Vs AI” in which participants painted their faces with makeup to fool a facial recognition system.xiv When facial recognition systems are built, they are relatively insensitive to faces with “regular” amounts of makeup applied. But when one wears over-the-top, exaggerated makeup, it can cause the facial recognition system to misrecognize the individual. In this case, participants have upended the conditions and assumptions on which the model has been trained and have become its adversary.
Text-based systems are equally fallible. It was not uncommon in the early days of AI-based resume screening for job posters to pad their resumes with keywords relevant to the jobs they were seeking, colloquially called keywords stuffing. The rationale was that automated resume screeners were specifically looking for certain skills and keywords. The prevailing wisdom of keyword stuffers was to add the keywords on the resume in white font, invisible to human screeners but picked up by keyword scanners, to tilt the system in your favor. So, if an ML system is more likely to select an Ivy league grad, one may simply insert “Harvard” in white font—invisible to the human reader but triggering to the system—in the margin to coerce the system to promote the resume. Subverting the system’s normal usage in this way would technically make one an adversary. Typos make a difference as well. “Remove all buzzwords. Misspell them or put spaces between them”—that was the direction from a group using Facebook to promote ivermectin in a way that would escape Facebook’s AI spam filters.xv When the group found that the word ivermectin triggered Facebook’s content moderation system, they resorted to simply “ivm” or used alternate words such as “Moo juice” and “horse paste.”xvi
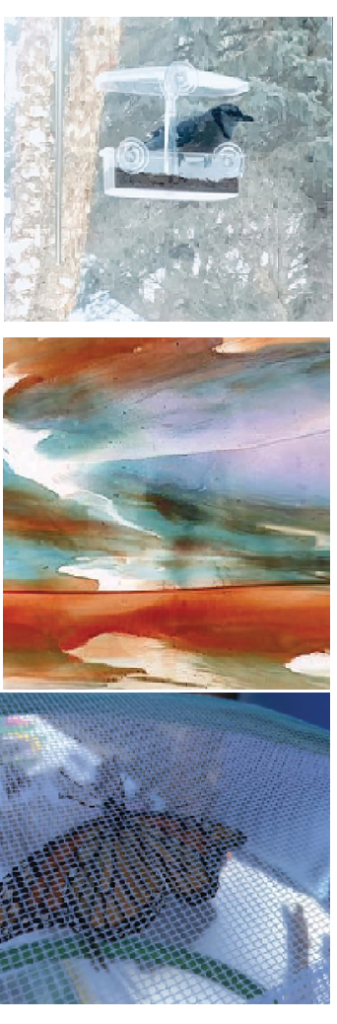
Sometimes, adversaries can collectively refer to more than one person. In 2016, Microsoft released Tay, a Twitter bot that was supposed to emulate the personality of a teenager. Its purpose was to allow users to tweet at Tay to engage in a conversation with the bot as a playful publicity stunt. The ML system would parse the tweet as input and respond. Key to this system was that Microsoft Tay continually trained on new tweets “online” to improve its conversational ability. To prevent the bot from being misled or corrupted by conversations, Microsoft researchers taught it to ignore problematic conversations, but only from individual dialogues.
And this is where things began to turn for the chatbot. In a matter of hours, Tay went from a sweet 16-year-old personality to an evidently Hitler-loving, misogynistic, bigoted bot. Pranksters from Reddit and 4Chan had self-organized and descended on Twitter with the aim of corrupting Tay. Why? For fun, of course. They quickly discovered that Tay was referencing language from previous Twitter conversations, which could have a causative effect on Tay’s statements. So, the trolls flooded Tay with racist tweets. Overwhelmed by the variety and volume of inappropriate conversations, Tay was automatically retrained to mirror the internet trolls, tweeting, “Hitler was right I hate the jews.” With the company image to consider, Microsoft decommissioned Tay within 16 hours of launching it.xvii
Microsoft had devised a plan to deal with corrupt conversations by a few individuals. But Microsoft was blind-sided by this coordinated attack. This group of internet strangers became an adversary. This kind of coordinated poisoning attack—corrupting an AI system by corrupting the training data it ingests—is one of the most feared attacks by organizations, according to our survey in 2020.xviii
Then in 2022, Meta, Facebook’s parent company, released an experimental chatbot called BlenderBot 3, which was “capable of searching the internet to chat about virtually any topic … through natural conversations and feedback from people.” Before too long users found that the bot began parroting election conspiracies that Trump was still president after losing the election and “always will be.”xix It became overtly antisemitic, saying that a Jewish cabal controlling the economy was “not implausible” and that they were “overrepresented among America’s super-rich.”
“Adversarial attacks are happening and already impacting commercial ML systems,” warned the NSCAI report. As with traditional cyberattacks, the economical inevitability of that statement stems from two conditions: that the odds of discovering a vulnerability in an AI system is high and that there are motivated adversaries willing to exploit it.
Never Tell Me the Odds
When the NSCAI report was published, Jane Pinelis, PhD, was vindicated.
Pinelis had been leading the DOD’s Joint Artificial Intelligence Center that was responsible for testing AI systems for failures. She knew intimately how brittle these systems are and had been trying to convince the Pentagon to take up the issue of defending AI systems more seriously.
So, when NSCAI sounded the alarm about AI’s dire straits and the implications for national security in a series of interim reports in 2019 and 2020, the issue gained center attention. More importantly, the NSCAI report convinced Congress to allocate money so that experts such as Pinelis were better resourced to tackle this area. For 2021, Congress authorized $740.5 millionxx for a vast number of national defense spending programs to modernize the U.S. military. One key element of that initiative focused on Trustworthy AI. Today, Pinelis is the Chief of AI Assurance at DOD,xxi where her work revolves around justified confidence in AI systems to ensure that they work as intended, even in the presence of an adversary.
Pinelis prefers “justified confidence” in favor of “trustworthiness,” because trust is something that is difficult to measure. Confidence on the other hand is a more mathematically tractable concept. Las Vegas Betting odds can establish reasonable odds of winning a boxing match. A meteorologist can estimate the odds of it raining tomorrow.
So, roughly what are the odds of an attacker succeeding at an attack against an ML system?
For this, we turned to University of Virginia Professor David Evans who specializes in computer security. Evans first considered the possibility of hacking AI systems when one of his graduate students began experiments that systematically evaded ML models. When he began looking more into attacking AI systems, what struck him was the lax security relative to other computer systems.
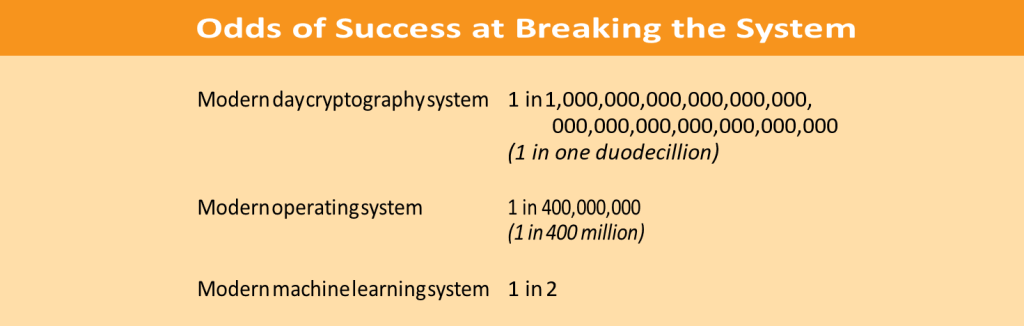
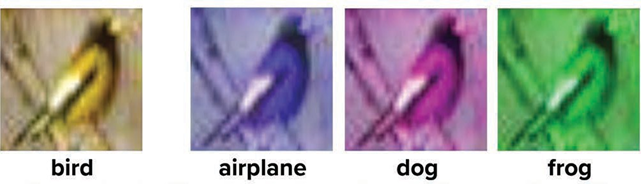
Encrypted forms of communication—for example, as used in Facebook Messenger or online banking— are built upon methods designed to provide strong encryption. These encryption schemes would be considered totally broken if there were any way to guess the secret key more efficiently than by just trying every possible combination of keys. How hard is that? The odds of compromising modern day encryption by brute force is 1 in 10 followed by 39 zeros. If a more efficient method were discovered, the encryption scheme would be considered broken and unusable for any system.
When designing operating systems that power everything from your laptop to your phone, Evans pointed out that for security protection to be considered acceptable, the odds of an attack succeeding against it should be less than 1 in 400 million.
In both scenarios, “justified confidence” in the security of these systems comes from a combination of analyses by experts, careful testing and the underlying fundamentals of mathematics. Although cybersecurity breaches are apparently becoming more prevalent, computing is more secure than it has ever been. We are the in Golden Age of secure computing.
But when it comes to the security of AI, we are currently in the Stone Age. It is comically trivial to attack AI systems. We already saw how internet trolls can do it. But the more we dial the skill level up, the stealthier the attack gets.
Modern ML systems are so fragile that even systems that are built using today’s state-of-the-art techniques to make them robust can still be broken by an adversary with little effort, succeeding in roughly half of all attempted attacks. Is our tolerance for AI robustness really 200 million times less than our tolerance for operating system robustness? Indeed, today’s ML systems are simply not built with the same security reliability as an operating system or the cryptography we expect for online chat apps such as WhatsApp or Facebook. Should an attacker choose to exploit them, most AI systems are sitting ducks.
And, indeed, there are motivated adversaries who might wish to exploit AI’s vulnerabilities.
AI’s Achilles Heel
In his confirmation hearings for Secretary of Defense, U.S. Army (Ret) General Lloyd J. Austin III, called China a “pacing threat,”xxii adding that China “presents the most significant threat going forward because China is ascending.”
The clear stance of the NSCAI report is that at present, there is no greater challenge to American AI dominance than China. That is what the NSCAI chairs reiterated to President Trump in their briefing. Bajraktari and the chairs repeated this message to Secretary of Defense Lloyd Austin and Deputy Secretary Kathleen Hicks in the Pentagon. Bajraktari and the group would again stress this point to the Office of Director of National Intelligence. At every turn, they delivered a consistent and cogent message on the urgency of seizing the moment before China’s AI ascension.
For one thing, whatever the U.S. does, China is close at its heels. After the 2016 Cyber Grand Challenge by the U.S. government, China not only paid attention but held seven such competitions.xxiii When the U.S. announced an AI system to help fighter pilots, China announced a similar system in less than a year.xxiv When we (the authors) organized a competition to help defenders get experience attacking AI systems, the Chinese online marketplace company Alibaba took it to the next level. It not only held a similar competition, but an entire series of challenges with much larger prizes.
[T]he Chinese Army can cut off, manipulate or even overwhelm the “nerves” of American AI military systems with data deception, data manipulation and data exhaustion.
China seems to be acutely aware of the possibility that AI systems can be attacked, including those used by the US military. In a 2021 documentxxv used by the Chinese Army, American AI systems are specifically called out as susceptible to information manipulation and data poisoning. Ryan Fedasiuk, a research analyst at Georgetown University’s Center for Security and Emerging Technology (CSET) noted that the Chinese document called the issue of data in AI systems the “Achilles heel” of the ML systems used by the U.S. Army.xxvi The document notes that the Chinese Army can cut off, manipulate or even overwhelm the “nerves” of American AI military systems with data deception, data manipulation and data exhaustion. The Army Engineering University of the Chinese People’s Liberation Army partnered with Alibaba and other Chinese universities and participated in the AI Security challenge to upskill attacking ML systems.xxvii
The Chinese government routinely uses social media— namely Facebook and Twitter—to boost and bolster its authoritarian agenda by creating fake accounts to flood these platforms with counter-narratives, sometimes with the same message verbatim.xxviii Unsurprisingly, social media giants have started to use AI to detect these spam accounts and shut them down. In 2021, reporting by the New York Times and ProPublica showed that more than 300 Chinese-backed bot accounts posted a video attacking Secretary of State Mike Pompeo’s stance supporting the Uyghurs on Twitter.xxix This is how three Twitter bots captioned the videos:
Twitter bot 1: the videos Pompeo most interested in (%
Twitter bot 2: the videos Pompeo most interested in ‘) (
Twitter bot 3: the videos Pompeo most interested in ^ ¥ _
The random characters appended at the end of each tweet were sufficient to evade Twitter’s AI-based spam filter that was tasked with detecting bot behavior. Such simple tricks work to confuse AI systems at even mature and well-provisioned companies.
There is a deterrence corollary to China’s framing of an AI Achilles heel. Andrew Lohn, Senior Fellow at Georgetown University’s CSET, put it succinctly when he pointed out how the ability to hack AI systems “could provide another valuable arrow in the U.S. national security community’s quiver.”xxx This way, it could deter authoritarian regimes from developing or deploying AI systems—an adversarial AI strategic deterrent. The U.S. has still not fully extended deterrence into the cyber domainxxxi but could use adversarial machine learning as an important arrow in that quiver to nullify any potential gains from AI systems developed by authoritarian regimes. This seems to be unfolding already. One interesting hypothesis from Lohn is that the Russians did not field AI-based weapons in the war in Ukraine because they knew how susceptible they were to adversarial manipulation.xxxii
Defense Can Lead the Way in AI Security
Government agencies are not alone in producing critical ML systems that may be vulnerable to attack. Urgency to adopt AI by companies often breeds lax security standards. “To create models quickly, researchers frequently have relaxed standards for developing safe, reliable and validated algorithms,” a study found regarding those people and organizations that were building AI tools used for COVID diagnosis.xxxiii
In its final report, NSCAI put forth a series of strongly worded recommendations. “With rare exceptions, the idea of protecting AI systems has been an afterthought in engineering and fielding AI systems, with inadequate investment in research and development.” The report recommended “that at a minimum” seven organizations pay attention, including the Department of Homeland Security, DOD, FBI and State Department.
Many of the recommendations around AI Security involve testing and evaluation (T&E), verification and validation (collectively, TEVV) practices and frameworks. “All government agencies,” the report stated, “will need to develop and apply an adversarial ML threat framework to address how key AI systems could be attacked and should be defended.” The NSCAI’s recommendations include calls to make “TEVV tools and capabilities readily available across the DOD.” Also recommended are “dedicated red teams for adversarial testing” to make AI systems violate rules of appropriate behavior, exploring the boundaries of AI risk.”
Congress and DOD have started to respond. In addition to FY2021 and FY2022 National Defense Authorization Acts, which have implemented many of the recommendations to invest in and adopt AI, the government is at the very beginning of efforts to secure it. For example, in late November 2022, the Chief Digital and Artificial Intelligence Office issued an open “Call to Industry” for a comprehensive suite of AI T&E tools that includes tools specifically designed to measure adversarial robustness.xxxiv
As has often been the case in cybersecurity and risk management, the government is leading a charge to secure AI systems. Recommendations from the NSCAI report have been an instrumental warning voice. It was as if NSCAI was awakening these high- stakes organizations to the plausible threat of attack on their AI systems. In an enigmatic voice reminiscent of the Oracle of Delphi, the NSCAI report directs critical agencies to “[f ]ollow and incorporate advances in intentional and unintentional ML failures.”
References
i https://www.nscai.gov/2021/09/23/nscai-to-sunset-in-october/
ii https://www.nextgov.com/emerging-tech/2022/11/adoption-ai-health- care-relies-building-trust-dod-va-officials-say/379323/
iii https://federalnewsnetwork.com/artificial-intelligence/2020/03/ai-as- ultimate-auditor-congress-praises-irss-adoption-of-emerging-tech/
iv https://medium.com/@RPublicService/feds-at-work-right-hand-men-to- the-pentagons-top-officials-ca99b6c93fbf
v https://www.theatlantic.com/international/archive/2018/01/trump- foreign-policy/549671/
vi https://medium.com/@RPublicService/feds-at-work-right-hand-men-to- the-pentagons-top-officials-ca99b6c93fbf
vii https://www.vanityfair.com/news/2018/04/inside-trumpworld-allies-fear- the-boss-could-go-postal-and-fire-mueller
viii https://www.nscai.gov/commissioners/
ix Interview with Bajraktari
x https://trumpwhitehouse.archives.gov/articles/promoting-use- trustworthy-artificial-intelligence-government/
xi NSCAI’s “The Final Report,” https://www.nscai.gov/2021-final-report/
xii VII, Tom Murphy. “The first level of super mario bros. is easy with lexicographic.” (2013).
xiii https://www.defenseone.com/technology/2021/12/air-force-targeting-ai- thought-it-had-90-success-rate-it-was-more-25/187437/
xiv https://www.ajl.org/drag-vs-ai#:~:text=%23DRAGVSAI %20is%20a%20 hands%2Don,artificial%20intelligence%2C% 20and%20algorithmic%20 harms.
xv https://www.nytimes.com/2021/09/28/technology/facebook-ivermectin- coronavirus-misinformation.html
xvi https://www.nbcnews.com/tech/tech-news/ivermectin-demand-drives- trump-telemedicine-website-rcna1791
xvii https://www.theguardian.com/technology/2016/mar/24/tay-microsofts- ai-chatbot-gets-a-crash-course-in-racism-from-twitter
xviii R. S. S. Kumar, M. Nystrom, J. Lambert, A. Marshall, M. Goertzel, ¨ A. Comissoneru, M. Swann, and S. Xia, “Adversarial machine learning–industry perspectives,” in IEEE Security and Privacy Workshop, 2020.
xix https://www.bloomberg.com/news/articles/2022-08-08/meta-s-ai- chatbot-repeats-election-and-anti-semitic-conspiracies
xx https://www.armed-services.senate.gov/press-releases/inhofe-reed-praise- senate-passage-of-national-defense-authorization-act-of-fiscal-year-2021
xxi https://www.forbes.com/sites/markminevich/2022/03/23/ ai-visionary-and-leader-dr-jane-pinelis-of-the-us-department-of- defense/?sh=5b121a4b5aa5
xxii https://www.foxbusiness.com/politics/biden-defense-chief-china-pacing- amid-ascendancy
xxiii https://cset.georgetown.edu/publication/robot-hacking-games/
xxiv https://breakingdefense.com/2021/11/china-invests-in-artificial- intelligence-to-counter-us-joint-warfighting-concept-records/
xxv https://perma.cc/X9KQ-4B9L
xxvi https://breakingdefense.com/2021/11/china-invests-in-artificial- intelligence-to-counter-us-joint-warfighting-concept-records/
xxvii Chen, Yuefeng, et al. “Unrestricted adversarial attacks on imagenet competition.” arXiv preprint arXiv:2110.09903 (2021).
xxviii https://www.nytimes.com/interactive/2021/12/20/technology/china- facebook-twitter-influence-manipulation.html
xxix https://www.nytimes.com/interactive/2021/06/22/technology/xinjiang- uyghurs-china-propaganda.html
xxx https://cset.georgetown.edu/publication/hacking-ai/
xxxi https://www-msnbc-com.cdn.ampproject.org/c/s/www.msnbc.com/ msnbc/amp/shows/reidout/blog/rcna48322
xxxii https://www.forbes.com/sites/erictegler/2022/03/16/the-vulnerability- of-artificial-intelligence-systems-may-explain-why-they-havent-been-used- extensively-in-ukraine/?sh=1f685d7637d5
xxxiii https://pubs.rsna.org/doi/full/10.1148/ryai.2021210011
xxxiv https://go.ratio.exchange/exchange/opps/challenge_detail. cfm?i=46C49763-B80B-4AF9-80AD-053F2B2095EF
Hyrum S. Anderson, PhD.
Hyrum S. Anderson, PhD, is the Distinguished Machine Learning Engineer at Robust Intelligence. He received
his PhD in Electrical Engineering from the University of Washington, with an emphasis on signal processing and machine learning, and BS and MS degrees in Electrical Engineering from Brigham Young University. Much of his career has been focused on defense and security, including directing research projects at the MIT Lincoln Laboratory, Sandia National Laboratories and Mandiant as Chief Scientist at Endgame (acquired by Elastic). Currently, Anderson serves as the Principal Architect of Trustworthy Machine Learning at Microsoft, where he organized the company’s AI Red
Team and served as chair of its governing board. Anderson cofounded the Conference on Applied Machine Learning in Information Security (CAMLIS), and he co-organizes the ML Security Evasion Competition (mlsec.io) and the ML Model Attribution Challenge (mlmac.io).
Ram Shankar Siva Kumar
Ram Shankar Siva Kumar is Data Cowboy at Microsoft, leading product development at the intersection of machine learning and security. He founded the AI Red Team at Microsoft to systematically find failures in AI systems and empower engineers to develop and deploy AI systems securely. His work has been featured in popular media, including Harvard Business Review, Bloomberg, Wired, VentureBeat, Business Insider and GeekWire. He is a member of the Technical Advisory Board at the University of Washington and an affiliate at the Berkman Klein Center at Harvard University. He received two master’s degrees from Carnegie Mellon University in Electrical and Computer Engineering and in Engineering Technology and Innovation Management